- The Process
- But, First: Lossy Versus Lossless Formats
- Acrobat Xs Image Exports
- And the Winner Is
But, First: Lossy Versus Lossless Formats
In choosing which image export format to use, you should consider whether the format you are considering is lossy or lossless. These terms describe the compression method used by the image format, and the difference between them can have a very big effect on the size and usability of your image file.
Lossless Compression: Good, But Big
A compression method is lossless if it can be uncompressed again to exactly the same data as was in the original image. The simplest such compression method is something called run-length encoding (often abbreviated RLE), which replaces the original image data with alternating lengths and values of repeating runs of bytes.
Eh?
Okay, consider the string of bytes depicted at the top of Figure 4. Note that there are a lot of repeating values in this data: a run of 63s, some 28s, etc. What RLE does is replace each repeating run of bytes with two (usually) values: the length of the run and the value of the repeating bytes, as in Figure 4’s lower graphic. The compressed data says the original consisted of nine 63s, followed by five 28s, a single 121, and so forth. The 18 bytes of data in Figure 2 became 8 bytes of compressed data.

Figure 4 RLE compression replaces each set of identical bytes with two values: the length of the set and the value of the bytes in that set. This is lossless, because it’s possible to resurrect the original data exactly.
What makes this “lossless” is that the compressed data has all the information needed to exactly reproduce the original image data; no information is ultimately lost in the compression process.
Of course, RLE compression only works with data that has repeating values, the more the better; for images, this generally means strictly monochrome data, black and white only. Other, more sophisticated lossless formats, such as LZW, can get good compression on a broad range of images.
The important point here is that if you stick to a lossless image format, you will never lose any image data; the image will never look worse than it started.
So, if lossless images look so good, why don’t we just compress everything with LZW or RLE and go on with our lives? Well, because you can make color and grayscale images smallermuch smallerif you can nerve yourself to throw away most of the image data; here we’re talking lossy compression.
Lossy Compression: Small, But Warrants Caution
Lossy compression works, in part, by analyzing the image and figuring out how much of its data can be discarded and still re-create a good likeness of the original image. Lossy image files, such as JPEG, can be very much smaller than their lossless brethren, but the uncompressed image is never exactly the same as the original.
As a crude example, consider the data diagrammed in Figure 5. The top line shows a row of pixels within the image. A lossy compression method might discard all this data except three pixels: the leftmost, middle, and rightmost pixel. This yields pretty good compression; our 17 pixels have been reduced to 3, about 18 percent of the original size. To display the image, the uncompression software would interpolate among the saved data, re-creating the colors of the pixels that were discarded, as in the bottom row in Figure 5.
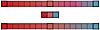
Figure 5 A lossy compression method extracts a sample of the image data and discards the rest. Here, our “compressed” data contains only 3 bytes of the original 17. Uncompressing this data results in a set of bytes interpolated from the values we retained; the resulting color values will be close to, but not quite the same as, the original. By the way, JPEG and other real-life lossy compression methods are way more sophisticated that my illustration here.
So, what do we gain? Tiny size. It’s amazing how small you can make an image by simply throwing enough of it away.
What we lose, of course, is fidelity. As you can see from Figure 5, the interpolated data won’t exactly match the original. You can get more accurate results by retaining a larger fraction of the original data, but then your image won’t compress as much. Furthermore, lossy algorithms tend to be especially bad at sharp edges within the image, giving rise to the familiar “artifacts” we’ve all seen within excessively-compressed JPEG images(Figure 6).
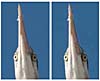
Figure 6 A close-up of an original image (left) and a low-quality JPEG version shows the artifacts to which JPEG images are prone. I hasten to point out that this JPEG image was purposely exported at the lowest quality setting (technically called “ick.”)
Most applications give you some control over this trade-off between image size and quality, usually a pop-up menu or set of radio buttons labeled “Quality,” as in Figure 7. The higher the quality you choose, the more of the original image data the software will retain when doing the compression and, therefore, the larger the final file size.
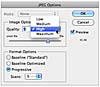
Figure 7 Most image-editing software gives you a chance to specify the quality of JPEG images; the higher the quality, the more of the original image data the compression process will retain.